Examples of Machine Learning in Education
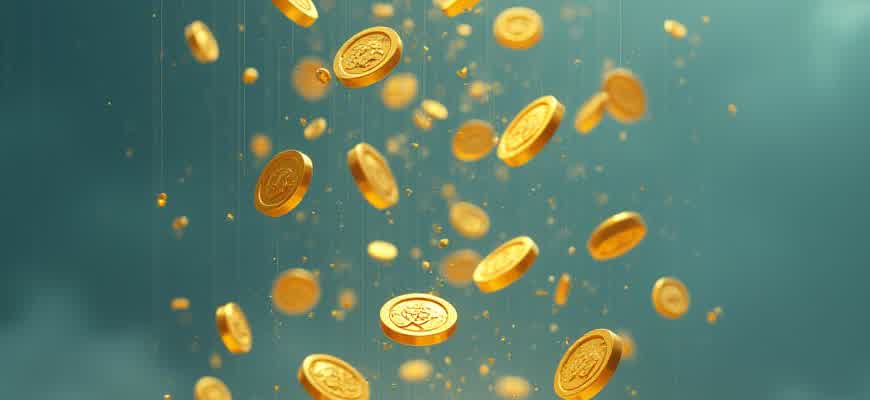
Machine learning is transforming the educational landscape by offering personalized learning experiences, improving administrative processes, and enhancing student outcomes. Below are some prominent examples of how this technology is being integrated into educational settings:
- Personalized Learning Systems: Machine learning algorithms adapt to the individual needs of students, tailoring lessons and exercises based on performance data. This helps in catering to diverse learning speeds and styles.
- Automated Grading: ML systems can grade assignments and exams, especially for subjects like mathematics and multiple-choice questions, reducing the workload of educators and providing instant feedback to students.
- Predictive Analytics: Data-driven predictions help identify students at risk of underperforming, allowing for early interventions to support them.
Key Benefits:
"Machine learning can assist in identifying patterns in student behavior, predicting future learning needs, and optimizing educational strategies."
Here are some specific uses:
Application | Description | Example |
---|---|---|
Smart Tutoring Systems | Provide real-time, personalized tutoring based on student input. | Carnegie Learning's MATHia software |
Adaptive Learning Platforms | Adjust the curriculum based on student performance and learning pace. | Duolingo's language learning app |
Student Performance Tracking | Monitor and analyze academic progress and behaviors. | Knewton’s adaptive learning platform |
Using AI to Tailor Learning Paths for Students
Artificial intelligence is rapidly transforming the education sector by enabling personalized learning experiences for students. By analyzing individual learning styles, strengths, and weaknesses, AI can create custom learning paths that adapt to each student’s unique needs. This allows for more efficient and effective learning, ensuring that students are engaged and able to progress at their own pace.
AI-powered tools use data such as past performance, behavior patterns, and even preferences to generate personalized content. These tools continuously assess students’ progress and make real-time adjustments, helping to keep them motivated and on track. Through this approach, students receive a learning experience tailored specifically to their current knowledge level and pace.
Key Benefits of AI in Personalized Learning
- Adaptive content delivery: AI ensures that content is presented at the right difficulty level, avoiding overwhelming or under-challenging students.
- Real-time feedback: Instant feedback helps students improve continuously and makes adjustments to learning paths as needed.
- Scalability: AI allows for scalable personalized education that can be applied to large numbers of students without losing quality.
How AI Adapts Learning Paths
- AI systems track individual performance on assessments and activities.
- It identifies knowledge gaps or areas of strength and recommends specific resources or exercises.
- Students receive a personalized learning path that continuously adjusts based on their progress.
Example of AI in Personalized Learning Paths
Feature | Traditional Learning | AI-Driven Learning |
---|---|---|
Content Delivery | One-size-fits-all | Adapted to individual needs |
Feedback | Delayed, periodic | Immediate, continuous |
Engagement | Fixed pacing | Flexible pacing |
"AI-driven learning pathways offer an unprecedented opportunity to meet the diverse needs of students, helping them achieve their full potential."
AI-Driven Assessment Tools for Real-Time Feedback
Artificial Intelligence (AI) is revolutionizing educational assessment systems by offering real-time, data-driven insights into student performance. These tools analyze responses immediately, providing valuable feedback that guides both students and instructors. Unlike traditional methods, AI systems can evaluate a wide range of metrics, including accuracy, speed, and understanding, allowing for more nuanced assessments.
One of the key advantages of AI-driven tools is their ability to continuously monitor progress and adjust difficulty levels dynamically. This approach helps to create personalized learning experiences, ensuring that each student is appropriately challenged. Below are some examples of how AI is enhancing assessment in education:
Benefits of AI Assessment Tools
- Instant Feedback: Students receive immediate feedback, which accelerates the learning process and reduces the time spent on misunderstanding concepts.
- Personalization: AI systems adapt to individual learning styles and needs, offering tailored exercises and assessments.
- Data-Driven Insights: Teachers gain valuable data on student performance, which helps in identifying areas for improvement.
Key Components of AI Assessment Systems
- Natural Language Processing (NLP): Used to evaluate written responses, helping AI understand and assess the context of students' answers.
- Machine Learning Algorithms: These algorithms predict student performance and adapt the difficulty level of assessments accordingly.
- Real-Time Analytics: Enables teachers to track progress and adjust teaching strategies based on up-to-date performance data.
"AI tools provide educators with the ability to assess more accurately and efficiently, fostering a more personalized learning environment."
Example Assessment Framework
Assessment Aspect | AI Functionality |
---|---|
Student Understanding | AI analyzes responses and provides immediate corrections or explanations. |
Progress Monitoring | Machine learning models track student improvement and identify areas needing attention. |
Personalized Challenges | AI adjusts the complexity of tasks based on individual performance trends. |
How Machine Learning Enhances Learning Outcomes through Adaptive Testing
Adaptive testing leverages machine learning algorithms to tailor assessment experiences to individual students' abilities. By analyzing performance in real-time, these systems adjust the difficulty of questions based on previous responses, creating a more personalized and efficient testing environment. This dynamic approach allows for a more accurate measurement of a student's skill level compared to traditional static tests.
Machine learning models use data from past interactions to identify patterns and predict the areas where a student may need additional support. This enables educators to provide targeted feedback, ensuring that learning gaps are addressed effectively. Over time, these adaptive systems become increasingly adept at understanding a learner’s progress and adjusting accordingly.
Key Advantages of Adaptive Testing in Education
- Personalized Learning Experience: Adaptive tests adjust based on individual performance, offering a more customized approach to assessment.
- Faster and More Accurate Results: By focusing on areas that need improvement, adaptive testing can provide immediate feedback, reducing the time spent on irrelevant questions.
- Efficient Use of Resources: Students are not subjected to questions that are either too easy or too difficult, which optimizes both time and effort for educators.
How Adaptive Testing Works
- Initial Assessment: The student takes an initial set of questions, and machine learning algorithms analyze the responses.
- Dynamic Adjustment: Based on performance, the system selects the next set of questions, adjusting difficulty in real-time.
- Continuous Learning: Over multiple sessions, the system continues to adjust, improving the accuracy of assessments as more data is collected.
Benefits for Students and Educators
For Students | For Educators |
---|---|
Increased engagement through tailored experiences | Time-saving in grading and assessment |
Better understanding of strengths and weaknesses | More targeted interventions based on student needs |
Reduction in test anxiety with appropriately challenging content | Ability to track long-term student progress |
"Adaptive testing provides a dynamic and data-driven way to measure learning outcomes, creating a more efficient and personalized learning environment."
Enhancing Student Engagement through AI-Driven Gamification
AI-based gamification offers a powerful method for boosting student participation and interest in learning. By combining artificial intelligence with gaming elements, educational platforms create personalized and interactive experiences that can motivate students to stay engaged in their studies. This approach adapts to individual learning speeds, offering students a tailored experience while integrating elements of fun and challenge.
Through dynamic learning environments powered by AI, students are encouraged to complete tasks and missions, earn rewards, and level up, much like in video games. This method not only enhances motivation but also promotes deeper engagement, as students become more immersed in their learning journey. The use of AI allows for real-time feedback and adaptive challenges, ensuring that learners stay on track and face appropriate levels of difficulty.
Key Features of AI-Based Gamification in Education
- Personalized Learning: AI analyzes students' performance and adjusts game elements accordingly, offering customized challenges and rewards.
- Instant Feedback: Learners receive immediate insights into their progress, which helps them make quick adjustments to their approach.
- Progress Tracking: Gamified platforms provide visual representations of progress, motivating students to reach their goals.
"By gamifying education through AI, students not only learn more effectively but are also more likely to enjoy the process."
Examples of AI in Gamified Education
- Duolingo: An AI-powered language learning app that uses gamified challenges and rewards to keep learners motivated and engaged.
- Kahoot!: A quiz-based platform that incorporates AI to adapt questions to the student's knowledge level, increasing engagement.
- Classcraft: An educational game where students earn points for positive behaviors and achievements, driven by AI-driven assessments.
Benefits of AI-Driven Gamification
Benefit | Description |
---|---|
Increased Motivation | AI-powered rewards and progress tracking enhance student motivation and engagement in learning tasks. |
Adaptability | AI adjusts difficulty levels based on the learner's performance, providing a personalized experience. |
Improved Retention | Game-based mechanics keep students involved, helping them retain knowledge better than traditional methods. |
Automating Administrative Tasks with Machine Learning for Educational Institutions
Educational institutions are increasingly adopting machine learning technologies to streamline administrative processes, improving efficiency and reducing the workload on staff. These systems use data-driven approaches to automate routine tasks, allowing educators and administrators to focus more on core educational activities. Machine learning algorithms can handle tasks such as grading, attendance tracking, and student performance analysis, significantly reducing the time and effort required to manage these responsibilities.
By implementing AI-driven tools, educational institutions can optimize resource allocation, enhance communication, and provide more personalized support to students. Below are some key examples of administrative tasks that can be automated with machine learning:
Key Areas for Automation
- Grading Automation: Machine learning algorithms can grade assignments, essays, and exams by analyzing patterns in previous assessments. This helps save time for instructors while ensuring consistency in grading.
- Attendance Monitoring: Using facial recognition or behavioral data, machine learning systems can automatically track student attendance, alerting instructors about absenteeism without manual input.
- Student Performance Forecasting: Machine learning can analyze historical data to predict students’ academic performance, helping educators intervene early and provide necessary support.
Benefits for Educational Institutions
- Time Efficiency: Automating administrative tasks reduces manual effort, allowing staff to focus on higher-priority activities.
- Improved Accuracy: Machine learning algorithms are less prone to human error, ensuring more accurate and timely results.
- Personalized Support: Institutions can use predictive analytics to tailor interventions based on individual student needs.
Example of a Machine Learning Application in Administrative Tasks
Task | Machine Learning Application | Impact |
---|---|---|
Grading | Automated grading of essays and multiple-choice exams using NLP (Natural Language Processing) algorithms. | Reduces instructor workload, ensures consistency, and speeds up feedback for students. |
Attendance | AI-driven facial recognition or smartphone GPS tracking to record student presence. | Eliminates manual tracking and improves the accuracy of attendance records. |
Performance Prediction | Analyzing historical academic data to predict future student outcomes. | Allows early identification of at-risk students and enables targeted interventions. |
By automating routine administrative tasks, educational institutions can redirect their resources toward enhancing the learning experience, leading to more effective teaching and better student outcomes.
Predictive Analytics in Education: Identifying At-Risk Students Early
Predictive analytics is transforming the education sector by enabling institutions to proactively identify students who may be at risk of underperforming or dropping out. By analyzing historical data, machine learning models can detect patterns that are indicative of academic struggles. This early detection allows educators to intervene before issues escalate, offering students the support they need to succeed.
Through the use of predictive tools, schools can assess a wide range of factors such as attendance, grades, behavioral patterns, and engagement in extracurricular activities. By aggregating this data, institutions can create a more personalized learning experience and provide targeted interventions to improve student outcomes.
Key Factors in Predicting Student Success
- Attendance rates: Consistent absences can be a strong indicator of disengagement or personal challenges.
- Academic performance: Declining grades, especially in core subjects, can signal a need for immediate intervention.
- Behavioral trends: Changes in classroom behavior may point to emotional or social difficulties affecting academic progress.
- Engagement levels: Lack of participation in school activities or online learning platforms can reflect disengagement.
How Predictive Analytics Works in Practice
- Data Collection: Collecting student data from various sources like grades, attendance, and behavior records.
- Model Training: Using machine learning algorithms to analyze historical data and identify patterns of risk.
- Risk Identification: Generating early warnings for students showing signs of potential academic failure.
- Intervention: Offering personalized support, such as tutoring, counseling, or behavioral coaching, to at-risk students.
"By using predictive analytics, educational institutions can not only prevent academic decline but also foster a more supportive and inclusive learning environment for all students."
Example: Data-Driven Interventions
Student | Risk Factors | Suggested Intervention |
---|---|---|
John Doe | Declining grades, frequent absences | Personalized tutoring, engagement monitoring |
Jane Smith | Behavioral issues, low participation | Counseling, social skills development |
Tom Green | Low test scores, poor class attendance | Academic coaching, attendance tracking |
Natural Language Processing in Educational Content Creation
Natural Language Processing (NLP) is transforming the way educational content is created by automating and enhancing various stages of content development. It allows for the analysis and generation of text in ways that were previously labor-intensive, providing more personalized and adaptive learning experiences. NLP tools can be utilized to generate instructional materials, quizzes, and summaries, making educational content more accessible and tailored to learners’ needs.
By leveraging NLP, educational content creators can speed up content generation, improve text readability, and offer deeper insights into student performance. One of the key advantages of NLP in content creation is its ability to process and analyze vast amounts of text, providing meaningful feedback and recommendations to educators and learners alike. This can lead to more efficient learning experiences that are grounded in data-driven insights.
Applications of NLP in Content Development
- Automated Content Generation: NLP algorithms can automatically create quizzes, exercises, and even full lessons based on existing materials or predefined topics.
- Text Summarization: NLP techniques can condense long reading materials into concise summaries, allowing learners to grasp the key concepts quickly.
- Personalized Learning: By analyzing student responses and interactions, NLP can tailor content to meet individual learning styles and preferences.
Key Benefits of NLP for Educators
Efficiency: NLP tools can significantly reduce the time required to produce and adapt learning materials, allowing educators to focus on more impactful tasks.
Scalability: NLP-driven platforms can generate large volumes of content, making it possible to offer customized learning experiences to thousands of students simultaneously.
Challenges and Considerations
- Accuracy: While NLP systems have made significant advances, the complexity of human language can sometimes lead to errors in content generation and interpretation.
- Context Understanding: NLP models may struggle with understanding the context of certain terms, which can affect the quality and relevance of the educational material produced.
- Bias in Algorithms: NLP tools are only as good as the data they are trained on, and bias in training data can lead to biased content generation.
Examples of NLP Tools for Education
Tool | Application |
---|---|
Grammarly | Automated grammar checking and writing enhancement. |
ScribeAI | Summarization and paraphrasing of educational texts. |
Knewton | Adaptive learning system using NLP for personalized course recommendations. |
Machine Learning for Curriculum Design: Tailoring Content to Individual Needs
Machine learning (ML) has become a powerful tool in education, allowing for more personalized learning experiences. One of the key areas where ML is making an impact is in curriculum design. By analyzing vast amounts of data on student performance, ML algorithms can identify learning patterns, strengths, and weaknesses. This allows educators to create tailored content that addresses individual learning needs, ensuring that each student receives the appropriate level of challenge and support.
Machine learning can be used to adapt learning materials and resources dynamically, offering real-time adjustments based on student progress. Through continuous monitoring of student behavior and interactions, ML models can suggest the most effective learning paths. This level of personalization is not only beneficial for students but also helps educators in their decision-making process, making the curriculum more responsive and efficient.
Key Benefits of ML in Curriculum Design
- Personalized Learning Pathways: ML enables the creation of custom learning plans based on a student's unique abilities and progress.
- Real-Time Feedback: Continuous data analysis provides immediate insights, allowing for prompt intervention when a student is struggling.
- Adaptive Learning Materials: The content and difficulty level adjust dynamically to meet the changing needs of each learner.
"Machine learning can enhance the educational experience by continuously adapting the curriculum to meet the individual learning pace of each student."
Example of ML-Driven Curriculum Adjustments
Consider a learning platform that uses ML to adjust the complexity of math problems based on student performance:
Student Performance Level | Suggested Content |
---|---|
High performance | Advanced problem sets and real-world applications |
Medium performance | Intermediate problems with guided steps |
Low performance | Basic problems with additional explanations |